Researchers have mapped the evolution and process of natural selection of E. coli in order to gain a deeper understanding of antimicrobial resistance. These maps, called fitness landscapes, help better understand the step-by-step development and characteristics of E. coli resistance to eight different drugs—including antibiotics. Researchers hope their results and methods will be useful for predicting and controlling E. coli, and other bacteria, in the future.
The fitness landscape, the authors noted, represents the complex relationship between genotype or phenotype and a given environment. Previous studies have based fitness landscapes on mutational analysis, but they do not predict evolution for the whole cell. In order to address this problem, the new study inferred the phenotype-based fitness landscape by quantifying multidimensional phenotypic changes.
This work is published in PLoS Biology in the article, “Analysis of the evolution of resistance to multiple antibiotics enables prediction of the Escherichia coli phenotype-based fitness landscape.”
“The development of methods that could predict and control bacterial evolution is crucial to find and suppress the emergence of resistant bacteria,” said Junichiro Iwasawa, a doctoral student in the department of physics, Graduate School of Science, University of Tokyo. “Thus, we have developed a novel method to predict drug resistance evolution by using data obtained from laboratory evolution experiments of E. coli.”
The researchers used adaptive laboratory evolution (ALE) to “replay the tape” on the evolution of drug-resistant E. coli to the eight different drugs, gaining insight into what changes might occur to the bacteria during the longer-term process of natural selection.
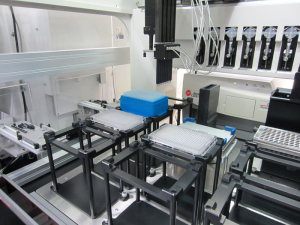
“While conventional laboratory evolution experiments have been labor intensive, we mitigated this problem by using an automated culture system that was previously developed in our lab. This allowed us to acquire sufficient data on the phenotypic changes related to drug resistance evolution,” explained Iwasawa. “By analyzing the acquired data, using principal component analysis (a machine-learning method), we have been able to elucidate the fitness landscape which underlies the drug resistance evolution of E. coli.”
Fitness landscapes look like 3D topographic maps. The mountains and valleys on the map represent an organism’s evolution. Organisms at the peaks have evolved to have better “fitness,” or ability to survive in their environment. Iwasawa explained, “The coordinates of the fitness landscape represent inner states of the organism, such as gene mutation patterns (genotypes) or drug resistance profiles (phenotypes), etc. Thus, the fitness landscape describes the relation between the inner states of the organism and its corresponding fitness levels. By elucidating the fitness landscape, the progression of evolution is expected to be predictable.”

The team believes the fitness landscapes it has mapped in this study, and the methods developed in the process, will be useful for predicting and controlling not only E. coli, but also other forms of microbial evolution. The researchers hope this will lead to future studies that can find ways to suppress drug-resistant bacteria and contribute to the development of useful microbes for bioengineering and agriculture.
Iwasawa concluded that “the next important step is to actually try using the fitness landscapes to control drug resistance evolution and see how far we can control it. This can be done by designing laboratory evolution experiments based on the information from the landscapes. We can’t wait to see the upcoming results.”