The use of artificial intelligence (AI) to develop new drugs is in its infancy. Nevertheless, AI-designed drugs have been entering the early phases of clinical trials over the past couple of years. GEN recently interviewed experts from four pioneering companies about how they are harnessing AI for drug discovery.
The potential of AI
“It is estimated that 90% of drugs fail in clinical trials,” laments Stefan N. Lukianov, CEO of Salve Therapeutics. Nonetheless, he is optimistic that failure rates will fall as drug development benefits from AI. “AI and machine learning,” he declares, “represent an exciting new way to improve efficacy and safety and get more drugs to the market.”
Lukianov explains that AI refers to the general ability of computers to operate in ways that resemble human intelligence. He adds that “machine learning is a subcategory of AI that uses preexisting data to devise novel solutions to complex problems.” More specifically, machine learning enables computers to identify patterns in data, make decisions, and learn without direct instruction.
He recognizes that the use of AI and machine learning in drug discovery is still in an early phase, but he expects that AI-based approaches will be better than traditional approaches. AI-based drugs, he explains, can be engineered to possess “efficacy features” such as affinity, target specificity, and optimized binding. He notes that wet lab validation can then be used to ensure that only the best candidates will proceed to clinical trials.
Salve Therapeutics: Exploring the human virome with AI
On the subject of the human virome, Lukianov notes, “Every tissue in the human body has a range of co-evolved viruses.” He adds that specific tissues are the locations of rare genetic diseases that can disrupt tissue structure and function. “Therefore,” he concludes, “naturally occurring viruses in these tissues are an ideal way to carry gene therapy payloads to treat the disease.”
Salve’s approach couples machine learning with computer-aided design (CAD). According to Lukianov, Salve’s CAD software is an engineering tool that allows users to model and simulate the properties and functions of an invention in a safe and inexpensive way. Also, the CAD approach can be combined with machine learning. Doing so, Lukianov says, enables virtual assessments of the “properties, outcomes, and risks of an invention through extensive, iterative analyses of various models and simulations.” He explains that this process uses preexisting data collected from similar inventions or contexts.
Salve is using its CAD approach to develop antibiotics in the form of phages—viruses that infect bacteria. “Each naturally occurring phage has evolved to target and destroy a specific bacterial species,” Lukanov says. “We are working to genetically engineer phages for greater potency, efficacy, and host range.” He expects phage antibiotics to improve the lives of transplant, burn, and immunocompromised patients.
Lukianov notes that phages are also being developed for anticancer and gene therapy applications. “Phages,” he emphasizes, “represent a versatile drug delivery mechanism that doesn’t harm human cells.”
Ultimately, Lukianov believes that the company’s products will be safe. “Phages only target bacteria,” he points out. “Therefore, they shouldn’t present a major risk to patients beyond a minor immune response due to the presence of foreign particles.” He also says it is possible to engineer antiviral agents to prevent unwanted replication, mutation, and spread of phages.
Biolexis Therapeutics: Using AI to target hot spots
David J. Bearss, PhD, the CEO of Biolexis Therapeutics, says that the company specializes in developing oral small molecules to target cancer and various metabolic, inflammatory, and neurodegenerative conditions. The company, he continues, is embracing the “potential of AI and empirical data to refine success rates, accelerate drug discovery, and advance medicine.”
Bearss notes that Biolexis’s technology is centered on its AI-based MolecuLern process. He says that MolecuLern has been trained on hundreds of 3D structures and screened against the company’s proprietary data library.
Biolexis provides services to a wide variety of clients—whose unique sets of empirical and wet lab data serve as training data for MolecuLern. “Ultimately, the goal is to discover targetable hot spots,” he declares. “We believe this approach is very beneficial over the hit-and-miss nature of traditional drug discovery.”
According to Bearss, MolecuLern’s success is due to the use of algorithms that integrate proprietary chemical library with experimental data. He stresses that this data-driven approach ensures that the molecules identified by MolecuLern are theoretically promising and have a solid foundation in real-world biology.
He notes that the MolecuLern process has led to the company’s pipeline of novel drugs, all discovered and developed in months instead of years. Indications include cancers and autoimmune, inflammatory, and metabolic disorders. Bearss highlights SLX-0528, currently in Phase Ib trials for pancreatic cancer. This drug was designed to control cellular differentiation, function, and interleukin release by T helper 17 cells, a subset of T cells characterized by the production of interleukin-17.
“MolecuLern is more than just a tool,” Bearss insists. “It is a paradigm shift in drug discovery. Whether you’re an academic researcher, a pharmaceutical company, or in the biotech space, MolecuLern empowers groundbreaking discoveries.”
BioSymetrics: Pioneering phenotype-driven approaches
Gabriel Musso, PhD, the co-founder and chief scientific officer of BioSymetrics, says that phenomics involves understanding the landscape of all potential phenotypes—defined as traits that can be observed. “Phenomics-driven drug discovery puts the disease phenotype at the center of the approach,” he elaborates. “The main goal is the reversal of the disease phenotype back to a healthy state through gene or small-molecule perturbation.”
The BioSymetrics approach is driven by its propriety Elion platform, which consists of three main components: vast amounts of clinical and genomic data, machine learning, and phenotypic experimental validation. In the first component, AI prioritizes genes responsible for causing disease phenotypes in experimental model systems.

The company then validates its findings using experimental phenotypic assays in zebrafish, which are transparent, grow quickly, and share 70% of their genes with humans. “Based on the genes prioritized in our data analyses, we use CRISPR gene editing to modify our zebrafish and validate the disease phenotypes,” Musso remarks. “From there, we will computationally prioritize gene targets.”
On the clinical side, BioSymetrics has worked on various clinical trials to improve inclusion criteria and identify biomarkers. For instance, the company worked with Johnson & Johnson on its vaccine clinical trials to predict disease severity in COVID-19 based on patient records at the time of diagnosis.
On the experimental side, the company has worked with pharmaceutical companies to experimentally test their drugs using genetic models of diseases. This allows for an understanding of which patients will respond to a given treatment, a key goal of precision medicine. Musso emphasizes that the Elion platform has enabled advances in pediatric epilepsy. These include the identification of key genes, the quantification of phenotypes, and the discovery of new hits and novel mechanisms of action.
NVIDIA: Harnessing the technology behind ChatGPT
Anthony Costa, PhD, is the global lead, life sciences developer relations, at NVIDIA. He points out that although generative AI is best known for producing text and images, it can do a great deal more. “ChatGPT really woke the world up to the power of generative AI,” he explains. “For example, virtually all drug hunters and developers are now considering how generative AI technologies can augment their drug platforms.”
Many generative AI tools are built on underlying AI models called large language models. “These large language models are improving the ability to predict drug properties and interactions,” Costa points out. “This has the potential to result in a higher quality of initial drug candidates.”
To help realize this potential, NVIDIA has developed BioNeMo, a cloud service for generative AI in biology that offers a variety of AI models for small molecules and proteins. “With BioNeMo, researchers and developers can leverage generative AI models with their proprietary data to rapidly predict the 3D structure and function of proteins and biomolecules,” Costa asserts. “This will accelerate the creation of new drug candidates.”
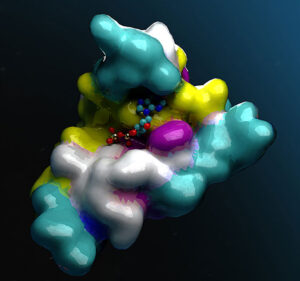
Costa relates that Evozyne, a Chicago-based startup company, recently used BioNeMo to engineer new proteins to treat phenylketonuria, a rare condition characterized by elevated levels of the amino acid phenylalanine. Lab tests eventually demonstrated that some of the AI-developed protein variants were more effective than natural forms.
BioNeMo has also been used by
Amgen to enhance the latter’s antibody design platform. Costa says that BioNeMo reduced the time required to build a custom large language model for antibody property prediction to just four weeks. In addition, Amgen leverages BioNeMo services to predict protein structures of generated candidate molecules. This has greatly enhanced Amgen’s ability to utilize AI tools for antibody discovery work.
Safety concerns with AI-developed drugs
Bearss emphasizes that various safety features are being integrated into AI-based drug development. For instance, Biolexis uses a variety of approaches—including a combination of traditional medicinal chemistry principles, advanced computational methods, and machine learning—to prioritize molecules with a high likelihood of safety. “Nevertheless,” Bearss remarks, “safety and potential unintended consequences of molecules developed by machine learning are important concerns that need to be addressed.”