A range of genetic factors can influence the onset of diseases like high blood pressure, heart disease, and type 2 diabetes, according to scientists. If we were to know how the DNA influences the risk of developing such diseases, we could shift from reactive to more preventive care, not only improving patients’ quality of living but also saving money in the health system.
However, tracing the connections between the DNA and disease onset requires solid statistical models that reliably work on very large datasets of several hundred thousand patients. Matthew Robinson, PhD, assistant professor at the Institute of Science and Technology (IST) Austria, together with an international team of researchers, has now developed a new mathematical model that could improve the predictive quality gained from large sets of patient genomic data and help develop personalized predictions about health risks, similar to what a physician does when discussing a family’s medical history.
Robinson and colleagues published their study “Genomic architecture and prediction of censored time-to-event phenotypes with a Bayesian genome-wide analysis” in Nature Communications.
“While recent advancements in computation and modelling have improved the analysis of complex traits, our understanding of the genetic basis of the time at symptom onset remains limited. Here, we develop a Bayesian approach (BayesW) that provides probabilistic inference of the genetic architecture of age-at-onset phenotypes in a sampling scheme that facilitates biobank-scale time-to-event analyses,” write the investigators.
“We show in extensive simulation work the benefits BayesW provides in terms of number of discoveries, model performance and genomic prediction. In the U.K. Biobank, we find many thousands of common genomic regions underlying the age-at-onset of high blood pressure (HBP), cardiac disease (CAD), and type 2 diabetes (T2D), and for the genetic basis of onset reflecting the underlying genetic liability to disease. Age-at-menopause and age-at-menarche are also highly polygenic, but with higher variance contributed by low frequency variants.
“Genomic prediction into the Estonian Biobank data shows that BayesW gives higher prediction accuracy than other approaches.”
The researchers selected several hundred thousand genetic markers, and using their statistical model, linked the composition of these markers to the onset of high blood pressure, heart disease, or type 2 diabetes in the patients in the database. The team was specifically interested in the patients’ age at disease onset. With this information, they can then use their model to predict probabilities for when a disease might occur.
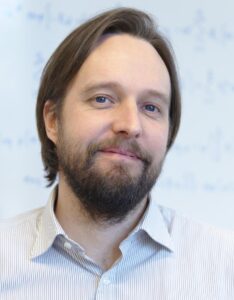
IST Austria
Yet, this statistical model cannot construct direct relations between certain genes and disease onset, but only provides an improved prediction of probabilities of disease onset. There is also an important difference between commonly used black-box models for big data studies and this method by Robinson and his colleagues: Black-box models produce predictions, but their inner workings cannot easily be understood by humans because of the many layers of abstraction they use. In contrast, the model by Robinson and his colleagues provides trackable statistical computations.
Using patient data
Being able to understand the inner workings of a mathematical model for producing predictions about health and disease onset is an important part of an ethical approach to using large sets of sensitive patient data. With this, the researcher can explain how the predictions were generated.
Harnessing the full potential of such predictive methods requires both effective models and the collection of large genomic datasets that comes with its own concerns of data security and privacy that both the researchers and the health care system have to address. Strict measures of data security have to be obeyed when using patient data. Only with the permission of the respective ethics boards, were the researchers able to access anonymized patient data from state-funded biobanks in both the U.K. and Estonia.
The scientists used the data from the U.K. to build their model and the data from Estonia to test its predictive power. The latter even produced some first personalized risk assessments of disease onset. These then will be relayed through the Estonian health care system back to the patients, giving them the incentive to take preventive steps.
The new statistical model by Robinson and colleagues is just one step towards using the full potential of large genomic datasets for preventive healthcare. Both the models and the data infrastructure of biobanks, together with a robust and secure data protection system, are needed to fulfill the promises of personalized predictive medicine.
“In general, whenever you visit a doctor they typically ask about your family history of particular diseases because family history is the leading risk factor for many common later-life diseases. However, this information is often incomplete,” Robinson tells GEN. “Predictions made from DNA are a better way of informing clinicians as to what the family history is of different people. They are a form of preventative medicine, whereby this information would be used to initiate screening for certain groups of people, or conveyed to patients in the hope that it may help them to make lifestyle choices.
“The evidence currently suggests that preventative medicine can save health systems money in the long term. I think genomic predictors, while poor at informing us individually of our specific risk, are able to identify groups of people who are at higher risk than the population average.”