Processes of drug discovery and the early stages of drug development remain enigmatic and rely heavily on experience-induced intuition and chance. Pharmaceutical companies have developed workflows that have proven expensive and lacked efficiency, resulting in tremendous overall costs in moving a candidate drug from the laboratory to the clinics.
AI (artificial intelligence)-based software solutions can offer a key ingredient—a substratum of connectivity—that drug companies often lack. The art of injecting such AI tools into existing workflows requires careful planning and a deep understanding of the industry’s management systems.
Nimble integrations
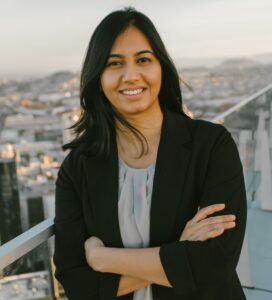
“In my experience, there are many experts at different levels of drug development but there is very little cross-collaboration. This creates inherent risks which result in a high failure rate in drug development pipelines. Companies die because they can’t meet the endpoint of the clinical trial and sustain the time and cost of the process,” says Jo Varshney, PhD, founder and CEO of VeriSIM Life.
The discovery and development of novel pharmaceuticals depend on transparent data sharing. However, the best of data sharing policies and practices may fall short of conveying critical insights among siloed departments.
“Our goal was to develop a straightforward and simple, nimble and agile workflow integration system,” says Varshney.
Flexibility is key in compiling and assimilating early-stage discoveries and closing the translational gap by moving the best possible candidate into the next phase of drug development. Key deliverables for AI solutions in the pharmaceutical industry include a quantifiable reduction in the risk of failure rates through the identification of dead-end investigations, experimental errors, and unnecessary assays, such as expensive and unnecessary animal studies.
BIOiSIM, developed by VeriSIM Life is a computational platform that functions as a virtual drug development engine. It integrates diverse workflows over the continuum from discovery to clinical trials. Repeated application of the system not only predicts efficiencies and risks in aspects of drug development but also generates recommendations for better options.
“Our hybrid AI system uses several machine learning models and quantitative methods that reflect the biological relevance, to address key questions,” says Varshney.
Keeping score
One of the problems of AI/ML-based tools is that outputs such as predictions are difficult to trace back to determine their strength. To circumvent this issue and offer clients an insight into the system’s predictions, BIOiSIM employs a translational index score, a concept similar to the FICO score used in financial predictions and credit card checks.
“We do not want to make our clients be AI/ML experts to be able to use or interact with our software. In fact, we want our clients to be experts in their assets. But we want them to know how well our methods work and how confident we are with our system,” says Varshney. “The score enables that and gives the transparency needed without the client having to become an expert in modeling, machine learning, or AI.”
An itemized breakdown of the translational index score provides customers insights on what is working, what needs improvement, and recommendations on how things can be done differently for better outcomes. This may include weighted measures of parameters such as the drug’s properties, animal study outcomes, and first-in-human doses.
“That’s the visibility we are providing so that you are not burned by the black box of unknown facts. It also helps you determine whether you want to do something different to improve the score and we also provide those recommendations,” says Varshney.
The translational index score also helps smaller biotech companies have an open discussion with larger companies that might be interested in acquiring assets or initiating partnerships.
“With TIS, you’re not only thinking about the financial risk, de-risking through risk-adjusted NPV [net present value], you’re also looking at de-risking the IP asset. That is a powerful combination and such a good and equitable strategy for any company to work. Then you’re not thinking about ML/AI, you’re thinking about what you need to do to evolve further and faster.”
Evolution not revolution
BIOiSIM focuses on the strategic use of AI solutions at key stages in the pipeline to integrate silos inherent in the drug development industry without overhauling established methods and starting anew based on an AI infrastructure.
“We want to build partnerships without you having to learn something completely new to use it. Being able to customize to fit into any workflow in any biotech company, makes us first-in-class,” says Varshney. “Because most tools and software out there are non-customizable, and non-scalable. They don’t use AI the way we do.”
Varshney illustrates the difference between existing AI tools for drug development and BioiSIM as the difference between the cruise control feature in existing cars and self-driving autonomous vehicles.
“The problem with cruise control is that it does not tell you what is ahead on the road. You have to constantly watch the road,” says Varshney, who intends to nurture the autonomous mindset in the pharmaceutical industry through AI-driven learning solutions and insights.
“Our solution offers evolution not revolution because pharma has been around for decades and they have a certain way of doing things,” says Varshney.
Digital transformation can start with uprooting the entire existing protocol established in the drug industry over time and use AI/ML to rebuild systems. Some experts and companies believe this is the ideal way forward. The alternative is to enable experts at these companies to transform their workflows to make them more efficient, reduce risk, time, and cost, empowering them to get the drugs to the market faster.
“We choose the latter way,” says Varshney. “We want to enable existing companies by showing them a better way that does not involve changing everything. You just have to integrate, you have to plug and play in different aspects to get the answers you need to move forward faster and have more visibility on risks before you spend millions of dollars. That’s a win-win situation for both our clients and us. It makes the interaction equitable.”
Varshney believes that though the industry is not there yet, AI-driven predictions might one day replace preclinical animal studies altogether.
“We want to use our system to tell you about wastage you can avoid,” says Varshney. The FDA modernization Act 2.0 which emphasizes the importance of non-animal tests is a step in the right direction, she notes.
Case study
“Our clients range from academic partners to large companies as well as hospitals. We have announced our partnership with the Mayo Clinic. Several of our clients’ compounds are now in Phase I trial based on the expertise, simulations, and predictions that we have provided,” says Varshney.
BIOiSIM has been used to inform personalized dosing of Chlorzoxazone (a muscle relaxant) in type 2 diabetes patients. Toward this end, the platform predicted pharmacokinetic (PK) profiles of subjects included in the study.
Physiological differences in patients can impact dosing regimens. Cohort variance based on disease condition or subject-specific characteristics, such as age, weight, gender, race, etc., can impact their PK and pharmacodynamic (PD) profiles. In such cases, stratified patient dosing may be required to correctly administer a safe and effective dose.1
For this project, BIOiSIM’s PK prediction model was trained and validated on over 1,000 compounds. Physiological parameters used in the model were extracted from published research and other credible sources. The platform simulated drug disposition in vivo in healthy subjects and compared this to the validated data.
According to published studies, hepatic clearance can affect a compound’s PK. The platform, therefore, identified subject-specific hepatic clearance that differs between healthy subjects and patients with type 2 diabetes and simulated outcomes in type 2 diabetics to capture the difference in PK disposition metrics.
With the overarching aim of creating medicines that are personalized for treatment the different applications of BIOiSIM’s AI-driven computational platform go beyond stratified dose optimizations to include optimizations for routes of administration, drug-drug interactions, combination therapies, efficacy, safety and toxicity profiling, and selection of animal and disease models.
Reference
1) Wang Z, Hall SD, Maya JF, et al. Diabetes mellitus increases the in vivo activity of cytochrome P450 2E1 in humans. Br J Clin Pharmacol. 2003;55(1):77-85.