
Scientists face slim odds when trying to turn a molecule into a medicine. Most studies put the batting average at about 0.100—or 1 in 10. Some go a little higher, some a little lower, but the success rate for drug discovery is never “good.” Some scientists believe that the success rate could be improved if drug discovery were to apply artificial intelligence (AI), that is, if it were to use advanced computational tools such as machine learning (ML) and molecular dynamics simulation. Besides leading to more medicines, AI might even allow the creation of better medicines.
Traditionally, a drug discovery project starts with basic research to uncover targets that may be susceptible to attack, such as a disease-related protein receptor on the surface of particular cells. Then, scientists use techniques like high-throughput screening to see which compounds bind the target. (These compounds can come from libraries of tens of thousands or even millions of molecules at large pharmaceutical companies.) After that, various methods of biological and chemical testing are used to fine-tune the structure or test other features, such as a compound’s ability to reach the target in an organism.
To some extent, AI can be used in all these steps. Essentially, AI looks for patterns in data that can be used to sharpen predictions about which compounds will become medicines. Some AI-based tools are already being applied to discovering tomorrow’s medicines. It remains to be seen how ready the industry is for this transition.
Top-rate targeting
When it comes to improving medicine with computational tools, one of the top players is GNS Healthcare. The company’s chief commercial officer Iya Khalil, PhD, says, “AI is being used to really leverage learning from large-scale datasets, and those large-scale datasets are used to get to better novel targets.” But she adds, “We’re still in the era of really trying to leverage what we can learn from genetic data and trying to understand what are the causal drivers of disease.”
The missing information about what drives a biological system—healthy or diseased—explains why a compound often fails in Phase II or III trials. With better knowledge—created from applying AI to whole-genome, phenotypic, and clinical data—scientists will find better starting targets, Khalil believes, “because you’re learning it directly from the human population.” Then, by turning off specific genes to see what changes, scientists may get even more data to dump into computational models.
Many techniques use AI like a black box: It finds patterns in the data, but no one knows what, if anything, they mean. Instead, Khalil prefers causal, or white box, AI. “We use it to get causality right from the beginning, and not just learn patterns,” she notes.
Still, making that work depends on lots of data, what Khalil calls deep data, such as a patient’s genomic and molecular data, and phenotypic and clinical data. Getting that data requires interdisciplinary teams. As an example, GNS Healthcare works with the Multiple Myeloma Research Foundation and others to collect more data. Khalil would like to work with hundreds of thousands of variables per person. It sounds like a lot, adding up to billions of data points, Khalil admits, but “it’s data that we can collect today.”
To put so much data to work in the best ways, Khalil and her colleagues do in-house development and coding, and run the simulations with cloud computing, like Amazon Web Services.
Seeing the sense
Data is so plentiful that scientists struggle to make sense of it. Alexis Borisy, executive chairman of the board at Celsius Therapeutics, says that the company analyzes tens of thousands of gene transcripts in cells from hundreds of human samples. In all, Celsius scientists work with a dataset with millions of dimensions. “That’s more data and dimensionality than you can look at and understand,” he says. “Machine learning helps you focus on the key things.”
No matter how sophisticated the AI might be, data fuels it. “Any learning system is only as good as the data you’re sending it,” Borisy insists. “There are plenty of anecdotes of deep learning finding patterns in the data that were total anomalies.”
By feeding computational techniques with real biological data, scientists at Celsius hope to find actionable information. “The beauty here,” observes Borisy, “is bringing the power of computation together with the power of biology and chemistry.”
Other companies also use AI to see more in disease-related data. At Gritstone Oncology, chief technology officer Roman Yelensky, PhD, and his colleagues analyze cancer-created neoantigens, which are peptides (protein fragments) from cancer-mutated genes that can trigger an immune system attack on the tumor. The idea is that all of the mutations in a patient’s tumor can be analyzed, and then AI can determine which ones to attack. By analyzing data derived from analyses of more than one million peptides that had studded the surfaces of hundreds of tumors, Yelensky and his colleagues created a training dataset.
Now, the company’s system can explore a person’s tumor for the top-20 candidate neoantigens to attack, out of 100–200 mutations. Building a cancer vaccine from 20 neoantigens is a balance, Yelensky explains: “With more neoantigens, you get more shots on goal, but you are using few enough to limit the odds of eliciting an irrelevant immune response.” The company is just starting a Phase I trial of this process, so the number 20 might change.
Other scientists also explore the role of proteins in diseases. Pat Walters, PhD, senior vice president of computation at Relay Therapeutics, and his colleagues are refining a system that runs thousands of molecular simulations and uses ML as part of an effort to improve the drug discovery process. Computational analysis of these simulations tracks a variety of parameters, such as how much a small molecule moves in a protein binding site and the stability of intermolecular interactions. By comparing the trajectories of active and inactive ligands, the system learns to predict if a novel compound is likely to bind to a particular protein. According to Walters, the trickiest part is “capturing the fundamental physics and expressing that in a form that can be processed by a machine learning program.” So far, Walters admits that it’s too early to tell how much better Relay’s approach will be than the traditional drug discovery process.
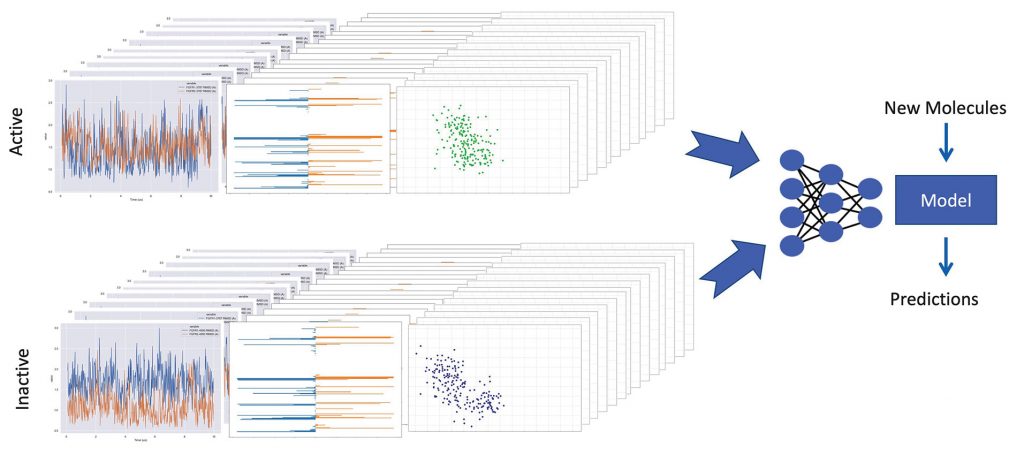
Making the transition
Just because using AI in drug discovery sounds like a good idea doesn’t mean everyone will agree. The methods of discovering drugs at pharmaceutical companies are pretty traditional. Also, many of the processes are familiar to regulators. Given the penchant to stick to standard approaches, it will take some time to prove the value of AI in drug discovery.
When asked about the current level of AI uptake in the drug discovery world, Khalil says, “It depends on the pharma companies—some have made a commitment to becoming more AI- and ML-driven, are quite serious about it, and are putting investment and teams behind it, and some aren’t ready yet.”
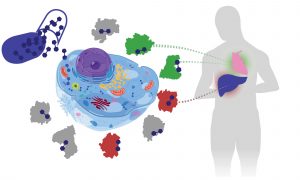
One science and technology company that is ready is Merck KGaA. Although Klaus Urbahns, PhD, head of discovery and development technologies at the biopharma business of that company, points out that early AI-based projects didn’t always produce useful results, the technology is rapidly advancing and looks too promising to deny it further investment and exploration. In December 2018, Urbahns and his colleagues announced a licensing agreement to use Ligand Express, which is a computation tool for proteome screening from Cyclica. Urbahns hopes that the results will “predict how molecules would interact with human biology.” He sees the results fitting best with early-stage drug discovery.
In creating Ligand Express, Cyclica CEO and president Naheed Kurji and his colleagues twisted the very basis of drug discovery. Instead of designing a single-target drug, scientists at Cyclica build ones that aim at multiple targets, which is called polypharmacology. By combining this approach with computational techniques, says Kurji, “We’re taking a more holistic view and thinking about all of the targets in
a cell.”
Ligand Express starts with a computational description of a small molecule, one with an atomic mass of just 200–900 Da. For comparison, aspirin is 180 Da. The format of the small molecule could be, for example, a simplified molecular-input line-entry (SMILE) string, which is a computer-friendly description of the molecule’s structure. Then, Ligand Express computes the list of targets likely to interact with this molecule, determines which interactions will turn the target on or off, and then links those reactions to downstream pathways to see how it might affect a person’s biology overall.
Through a web-based interface, a scientist can organize the data to examine the targets or see how the targets of pathway might play a role in a specific disease. “Then, only the targets linked to your disease area and that interact with your small molecule show up,” Kurji explains.
These processes depend on large databases and extensive computation—so large, in fact, that it used to take Cyclica about a month to screen 20–30 molecules against about 150,000 protein targets. Today, the deep learning tools from Cyclica can screen 10,000 molecules across the human proteome in less than two hours, Kurji says. Plus, this method shows scientists the interactions that are most likely to be on target, and weeds out most of the off-target effects. Out of all of the interactions indicated by the results, Kurji estimates, half of the top 60 will be true positives. “Compared to experimental results, that is equal, if not better,” he asserts.
Urbahns thinks of Ligand Express as another colleague. “Any scientist who has analyzed early datasets knows that it’s immature, and the next steps are uncertain,” he elaborates. “Individuals have a personal history that impacts the direction that a project will take, and it would help to have another voice or computer to validate a direction.” So far, though, Urbahns cannot provide an example of a drug discovered with AI. But he says, “I can see it happening one day.”
Even then, no drug is likely to come from AI alone. “What AI and machine learning promise to do is not magic,” Khalil explains. “It promises to help automate what we can learn from data, and I don’t think there is a pharma company out there that does not want that.”
Mike May ([email protected]) is a freelance writer and editor.